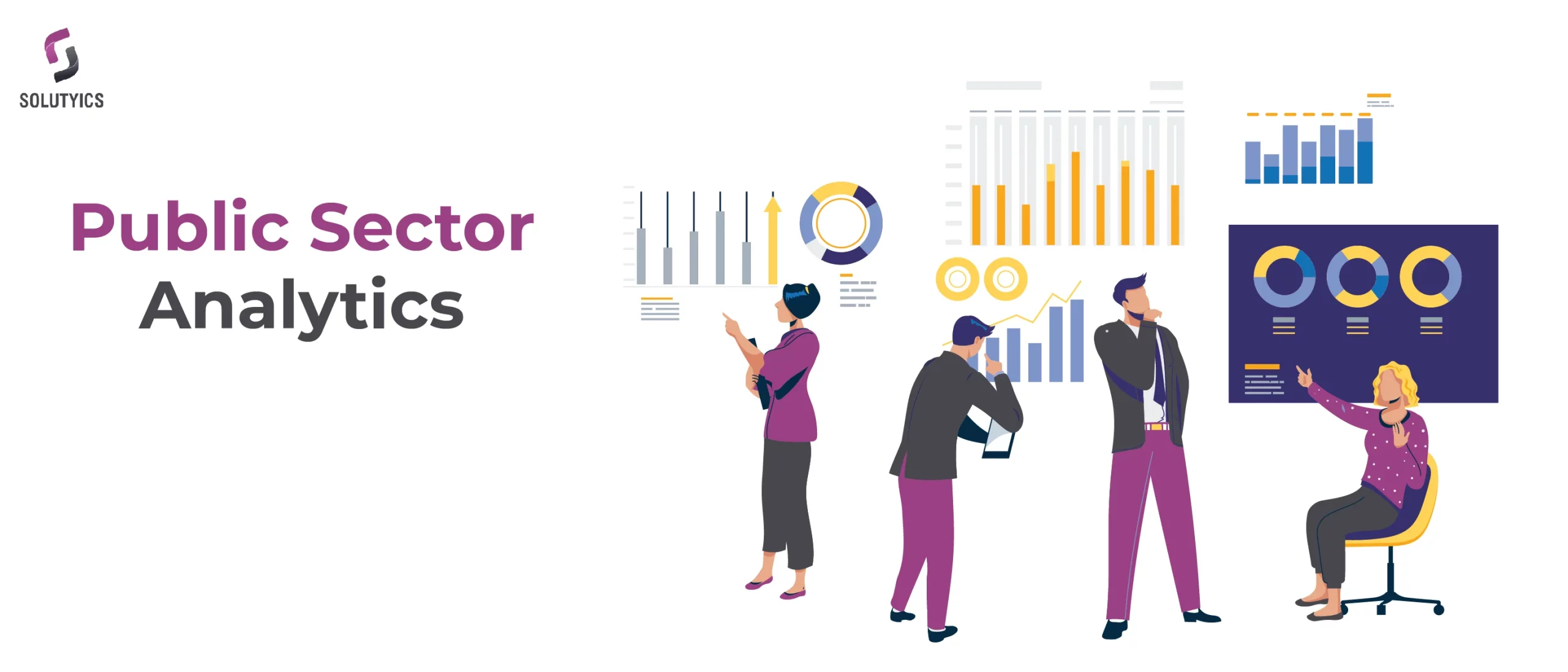
Public sector analytics refers to the use of data analysis and advanced analytical techniques to gather, process, interpret, and visualize information within the public sector. The public sector includes government agencies, departments, and other organizations that are funded by and serve the general public. Analytics in the public sector involves the systematic examination of data to extract meaningful insights, support decision-making, and improve overall governance and service delivery.
Public sector analytics can be applied across various domains, including healthcare, education, transportation, public safety, social services, and more. It enables government entities to make informed decisions, enhance transparency, improve citizen services, and optimize resource allocation. The use of analytics in the public sector has become increasingly important as governments seek to leverage data-driven insights for better governance and public service delivery.
Many countries around the world have adopted public sector analytics to varying degrees, using data-driven approaches to improve governance, enhance public services, and optimize resource allocation. Some countries have made significant strides in this area, realizing various benefits. Here are a few examples:
United States:
The U.S. government has been a leader in adopting analytics across different agencies. Initiatives like the Federal Data Strategy and the use of data analytics in healthcare through the Centers for Medicare & Medicaid Services (CMS) have aimed to improve service delivery, reduce costs, and enhance decision-making.
United Kingdom:
The UK government has embraced data analytics to enhance public services and policymaking. The Government Digital Service (GDS) has been involved in various projects, including data.gov.uk, which provides access to a wide range of government datasets. Analytics is used to improve efficiency in areas such as healthcare, education, and transportation.
Singapore:
Singapore has been a pioneer in using analytics to transform public administration. The government has implemented the Smart Nation initiative, incorporating analytics for better urban planning, transportation management, and public services. The use of analytics has contributed to Singapore’s reputation as a smart city.
Canada:
The Canadian government has integrated analytics into different departments and agencies to improve program effectiveness and policy development. For example, Statistics Canada uses analytics to gather, analyze, and disseminate statistical information, contributing to evidence-based decision-making.
Estonia:
Estonia is known for its advanced digital governance and has integrated analytics into various aspects of public administration. The X-Road platform facilitates data exchange between government agencies, and analytics is used to enhance efficiency, reduce bureaucracy, and improve citizen services.
Benefits of adopting Public Sector Analytics:
Improved Decision-Making:
Analytics enables governments to make data-driven decisions, leading to more effective and efficient governance.
Enhanced Service Delivery:
By analyzing data on citizen needs and preferences, governments can optimize the delivery of public services, providing more targeted and responsive solutions.
Resource Optimization:
Analytics helps in identifying areas for resource optimization, reducing waste, and improving the allocation of funds and personnel.
Increased Transparency:
Governments can use analytics to enhance transparency by providing citizens with access to data, making it easier for them to understand government actions and outcomes.
Better Policy Formulation:
Analytics supports evidence-based policymaking, helping governments formulate policies that are grounded in data and likely to have a positive impact.
Launching Public Sector Analytics in Developing Countries:
Integrating data and analytics into the public sector of your developing country requires a thoughtful approach. Here’s a guide accompanied by real-world examples:
Set Up a Solid Data System:
How:Conduct an in-depth analysis of existing data infrastructure. In India, the Aadhaar system is an example of a robust data system that efficiently collects and manages citizens’ demographic and biometric information, forming the basis for various public services.
Train Your Team:
How: Tailor training programs to the specific needs of your team. Rwanda, in its commitment to digital literacy, initiated the “One Laptop Per Child” program to provide teachers and students with the necessary skills for utilizing technology and data effectively.
Protect People’s Data:
How: Implement stringent data security measures. Singapore, with its Personal Data Protection Act (PDPA), ensures the responsible handling of personal data by organizations, safeguarding the privacy of its citizens.
Get Government Backing:
How: Advocate for the adoption of policies that support data-driven decision-making. The United Kingdom’s Government Digital Service (GDS) is a prime example, leading initiatives to transform government services through the use of data and technology.
Team Up with Others:
How: Foster collaborations with businesses, NGOs, and academic institutions. Kenya’s partnership with mobile service providers for initiatives like M-Pesa showcases how public-private collaboration can drive innovative data-driven solutions for financial inclusion.
Start Small, Learn Big:
How: Begin with pilot projects. In Brazil, the “DataSUS” initiative started small by digitizing health data. It evolved into a comprehensive system, providing valuable insights for healthcare decision-makers.
Share the Knowledge:
How: Establish open data platforms. The Open Data Portal in Mexico allows citizens access to a wide range of government datasets, promoting transparency and encouraging creative use of data for societal benefit.
Live and Breathe Data:
How: Integrate data into everyday decision-making processes. The Data-Driven Detroit initiative in the United States exemplifies how a city can embed data-driven practices to address urban challenges and improve public services.
Show Off Your Wins:
How: Celebrate successful analytics projects. In South Korea, the “Big Data Campus” showcases success stories, providing a platform for sharing insights and promoting the value of data analytics in government.
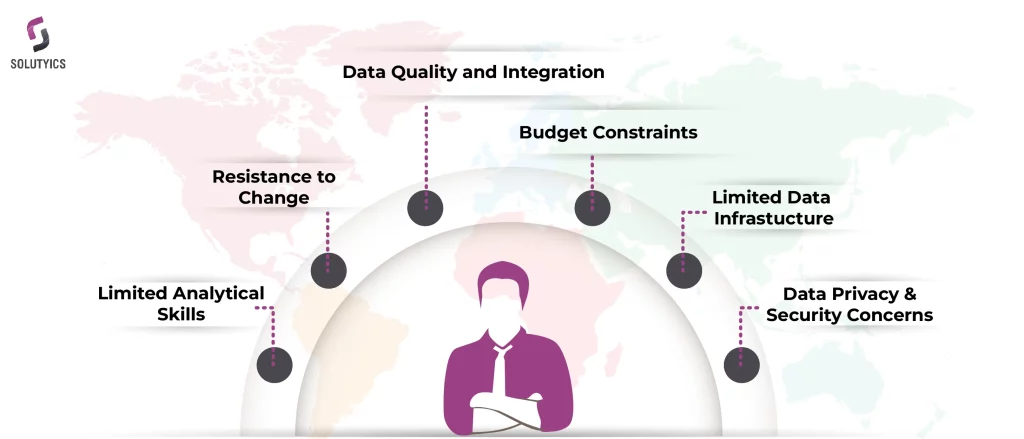
Challenges:
Adopting public sector analytics comes with various challenges, especially for developing countries. These challenges can vary depending on factors such as infrastructure, governance, and data management. Here are some common challenges faced by governments when implementing public sector analytics:
Limited Data Infrastructure:
Challenge: Insufficient data infrastructure, including outdated systems and inadequate storage capacities, can hinder the effective collection, storage, and processing of data.
Impact: Poor data infrastructure can lead to delays, inaccuracies, and the inability to harness the full potential of analytics.
Data Quality and Integration:
Challenge: Poor data quality, inconsistent formats, and a lack of standardized data across different government departments make it challenging to integrate and analyze information comprehensively.
Impact: Inaccurate or incomplete data can lead to unreliable insights, affecting the overall effectiveness of decision-making.
Limited Analytical Skills:
Challenge: The shortage of skilled professionals with expertise in data analysis and advanced analytics techniques poses a significant hurdle.
Impact: Without a skilled workforce, governments may struggle to extract meaningful insights from data and effectively utilize analytics for decision-making.
Resistance to Change:
Challenge: Resistance to adopting new technologies and data-driven approaches among government employees and stakeholders.
Impact: Cultural resistance can impede the integration of analytics into existing processes and hinder the overall effectiveness of data-driven initiatives.
Data Privacy and Security Concerns:
Challenge: Growing concerns regarding the privacy and security of citizen data can lead to hesitancy in implementing analytics initiatives.
Impact: Failure to address privacy and security issues can erode public trust and compromise the success of analytics projects.
Budget Constraints:
Challenge: Limited financial resources may impede the investment needed for infrastructure upgrades, training programs, and the implementation of advanced analytics solutions.
Impact: Insufficient budget allocations can slow down or even halt the progress of analytics initiatives.
Lack of Governance Frameworks:
Challenge: Absence of clear governance frameworks and policies for data management, sharing, and usage.
Impact: Without proper governance, there’s a risk of misuse of data, inconsistent practices, and challenges in ensuring data quality and integrity.
Complex Regulatory Environment:
Challenge: Navigating complex regulatory environments, including compliance with data protection laws and standards.
Impact: Failure to comply with regulations can result in legal challenges and hinder the adoption of analytics solutions.
Legacy Systems and Interoperability Issues:
Challenge: Dependence on outdated legacy systems and challenges in achieving interoperability between different systems.
Impact: Legacy systems may not support modern analytics tools, leading to integration challenges and limitations in leveraging new technologies.
Cultural and Organizational Challenges:
Challenge: Existing organizational cultures that do not prioritize data-driven decision-making can pose obstacles.
Impact: Overcoming cultural barriers and fostering a mindset shift towards embracing analytics can be a time-consuming process.
Addressing these challenges requires a holistic approach involving investments in technology, workforce development, and the establishment of clear policies and governance frameworks. Successful adoption of public sector analytics often involves overcoming these hurdles through careful planning, stakeholder engagement, and continuous improvement strategies.
Future of Public Sector Analytics:
The future of public sector analytics holds tremendous potential for transformative changes in the way governments operate, make decisions, and deliver services to citizens. Several trends and advancements are shaping the trajectory of public sector analytics:
Artificial Intelligence (AI) and Machine Learning (ML):
Future Trend: Increasing integration of AI and ML algorithms in public sector analytics for predictive modeling, automation of routine tasks, and more sophisticated data analysis.
Impact: AI and ML can enhance decision-making processes, automate repetitive tasks, and provide deeper insights into complex issues.
Predictive Analytics for Early Intervention:
Future Trend: The use of predictive analytics to identify potential issues before they escalate, allowing governments to take proactive measures.
Impact: Governments can anticipate and address problems in areas such as public health, infrastructure maintenance, and social services, leading to more efficient resource allocation.
Citizen-Centric Services:
Future Trend: Increased focus on personalized, citizen-centric services driven by analytics insights.
Impact: Governments can tailor services based on individual needs, preferences, and behavior patterns, leading to improved citizen satisfaction and engagement.
Open Data Platforms and Transparency:
Future Trend: Expansion of open data initiatives, providing citizens with increased access to government datasets.
Impact: Enhanced transparency, public accountability, and opportunities for citizens, businesses, and researchers to leverage data for various purposes.
Blockchain for Data Security and Transparency:
Future Trend: Exploration of blockchain technology for secure and transparent handling of government data.
Impact: Improved data security, reduced fraud, and increased trust in government processes through decentralized and tamper-resistant data management.
Integration of Real-Time Data:
Future Trend: Integration of real-time data streams for more dynamic and responsive decision-making.
Impact: Governments can quickly adapt to changing conditions, such as emergencies, traffic patterns, or public sentiment, leading to more agile governance.
Cross-Agency Collaboration:
Future Trend: Increased collaboration and data sharing between different government agencies and departments.
Impact: Breaking down silos allows for a more holistic view of issues, improved coordination, and comprehensive solutions to complex challenges.
Robotic Process Automation (RPA):
Future Trend: Adoption of RPA for automating repetitive tasks and processes within government operations.
Impact: Increased efficiency, reduced administrative burden, and cost savings in routine, rule-based tasks
Ethical and Responsible Data Use:
Future Trend: Growing emphasis on ethical considerations in data use, ensuring responsible handling of citizen data.
Impact: Enhanced public trust, compliance with data protection regulations, and a commitment to ethical practices in data-driven decision-making.
Geospatial Analytics for Urban Planning:
Future Trend: Leveraging geospatial analytics for comprehensive urban planning and development.
Impact: Improved city infrastructure, transportation systems, and disaster preparedness through location-based insights.
The future of public sector analytics will likely be characterized by a convergence of advanced technologies, a commitment to ethical data use, and a focus on citizen-centric services. As governments continue to invest in data-driven approaches, the potential for positive impact on governance, service delivery, and overall public well-being is substantial.
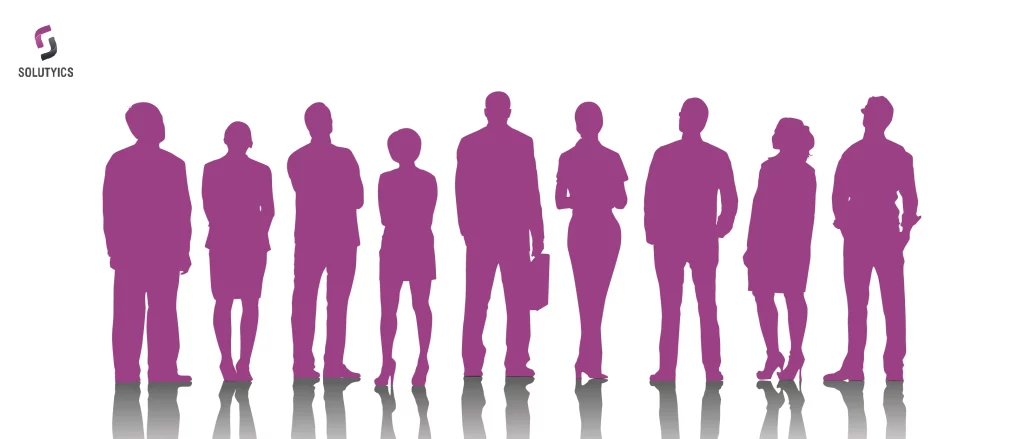
Conclusion:
In conclusion, the widespread adoption of public sector analytics is reshaping governance globally. Experiences from leading nations showcase the benefits: improved decision-making, enhanced service delivery, resource optimization, transparency, and evidence-based policy formulation.
Launching public sector analytics in developing countries requires strategic steps: establishing robust data systems, training skilled teams, safeguarding data, securing government support, fostering collaborations, and starting with pilot projects. Addressing challenges like limited infrastructure, data quality issues, and resistance to change demands a comprehensive approach.
The future holds promising trends, including AI and ML integration, predictive analytics, citizen-centric services, open data initiatives, blockchain for security, real-time data integration, cross-agency collaboration, RPA, ethical data use, and geospatial analytics. This convergence signals a transformative era, where technology and ethical considerations drive efficiency, transparency, and citizen-focused governance.
Author
Team Solutyics is a dynamic group of Analytics and AI specialists who bring together a rich mix of expertise. Their combined insights ensure that readers gain a deeper understanding of practical applications of Analytics and AI.