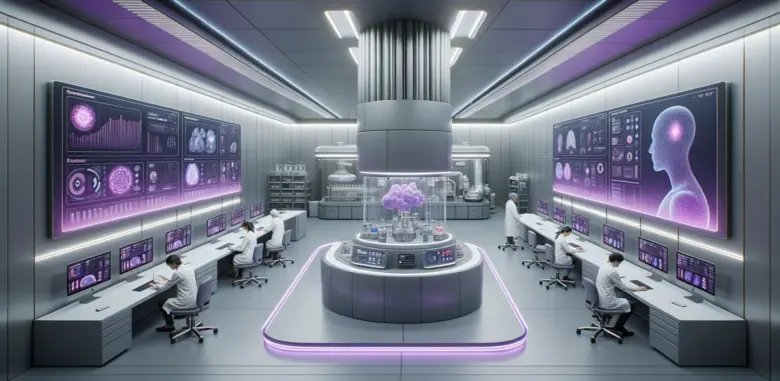
Introduction
Time changes, technology changes, and with the change in technology there always comes a great revolution in industries. Predictive analytics is an innovation in healthcare that will change and upgrade the healthcare sector. The transformation has caused a major shift in how medical professionals manage patient care, allocate resources, and improve their efficiency. Stay tuned because in this blog we will discuss its significance and benefits within the healthcare field.
What is Healthcare Predictive Analytics?
Analytics that predict outcomes in healthcare are known as Healthcare Predictive Analytics. Using statistical algorithms and machine learning techniques, healthcare predictive analytics is a data analysis field that forecasts patient behavior, healthcare trends, and future health outcomes. It relies on examining historical and real-time healthcare data.
It helps healthcare professionals make informed decisions and take useful steps to improve patient care, reduce costs of treatments, and optimize resource allocation.
It is not that easy. Healthcare predictive analytics requires tons of information such as medical records, test results, and even lifestyle details of a person to make predictions about their health. Collecting this much information is itself a very big challenge but the more the input is the better the output will be.
In the following sections, we will explain what can be achieved with healthcare predictive analytics.
Early Warnings:
Healthcare predictive analytics provides early warnings by analyzing a person’s health data over time. Interesting right? This is how it works:
Data Collection: Firstly, a lot of information about a person’s health is collected. This can include things like their medical history, lab results, lifestyle habits (like diet and exercise), and even genetic information.
Pattern Recognition: Special computer programs called algorithms go through all this data and look for patterns. For instance, they might notice that people who later develop diabetes often have certain blood sugar levels and lifestyle habits in common.
Prediction: Once these patterns are identified, predictive analytics can make predictions. For example, it might predict that someone has a higher risk of developing diabetes in the next few years based on their current data.
Alerting Healthcare Providers: These predictions are then shared with healthcare providers. If a person has a higher risk of a health issue, doctors can take action right now. They might recommend lifestyle changes, more frequent check-ups, or even specific treatments to prevent or manage the condition.
Through this doctors can spot potential health problems early, allowing for timely intervention and better health outcomes.
Saving Money:
One can save money by healthcare predictive analytics as “prevention is often cheaper than cure.” Are you wondering how?
Well, healthcare predictive analytics saves money by stopping potential health problems from becoming more severe and expensive. It’s like stopping a small leak in your house before it turns into a big flood, and this benefits both patients and healthcare systems.
Let’s see how it works:
Less Intensive Treatments: Treating a condition in its early stages typically requires less intense medical interventions, like taking pills or undergoing minor surgeries. These treatments are usually less expensive than complex surgeries or lengthy hospital stays.
Preventing Complications: By addressing health issues early, predictive analytics helps prevent complications. For instance, managing high blood pressure can prevent costly events like heart attacks.
Reduced Hospitalization: Early intervention can reduce the need for expensive hospitalization or emergency room visits, which are among the costliest aspects of healthcare.
Lower Long-term Costs: Preventing diseases from getting worse or managing chronic conditions effectively can significantly cut long-term healthcare expenses. Avoiding complications and costly treatments down the road results in substantial savings.
Smart Planning:
Hospitals can use healthcare predictive analytics for smarter planning by forecasting patient needs and ensuring they have the right staff and equipment available. To understand how it works read the following steps:
Data Analysis: Predictive analytics looks at historical data, like admission rates and patient patterns, along with other factors such as flu season trends and even local events. It identifies patterns and trends in this data.
Predictive Models: Special algorithms create predictive models based on these patterns. For example, it can predict that during a certain month, the hospital might see a higher number of patients with respiratory issues due to seasonal changes.
Resource Allocation: With these predictions, hospitals can plan ahead of time. They can schedule more doctors and nurses during busy times, make sure there are enough hospital beds, and ensure they have the necessary medical equipment ready.
Emergency Preparedness: Predictive analytics also helps hospitals prepare for emergencies. If it anticipates a spike in patients due to a disease outbreak, the hospital can stock up on medications, ventilators, and personal protective equipment in advance.
Efficiency: By allocating resources more efficiently, hospitals can reduce wait times, provide better care, and avoid overcrowding. This benefits both patients and healthcare providers.
Personalized Care:
Healthcare predictive analytics enables personalized care by tailoring medical treatment to each individual’s unique needs and health history. The following steps are involved in this process:
Risk Assessment: Based on the patterns in data, the system calculates a person’s individual health risk. For example, it might predict that someone has a higher risk of developing heart disease because of their family history and current lifestyle choices.
Personalized Recommendations: With this risk assessment, healthcare providers can offer personalized recommendations. These could include lifestyle changes (like diet and exercise), specific screenings, or targeted medical treatments.
Continuous Monitoring: Predictive analytics doesn’t stop at the initial assessment. It then continuously monitors a person’s health data, adjusting recommendations as needed. For instance, if someone’s risk for diabetes increases due to weight gain, the system can suggest changes to their treatment plan.
Better Outcomes: Personalized care ensures that patients receive treatments and interventions that are most likely to work for them. This increases the chances of successful outcomes and reduces the risk of adverse effects from treatments that might not be suitable for their unique situation.
As you have read earlier predictive analytics can streamline the healthcare industry making it more efficient. With early warnings and personalized care, it can save many lives and it can be used for the betterment of human beings. Moreover, it can save them money and enable hospitals to do smart planning ensuring they have the right staff and equipment available at the time of need.
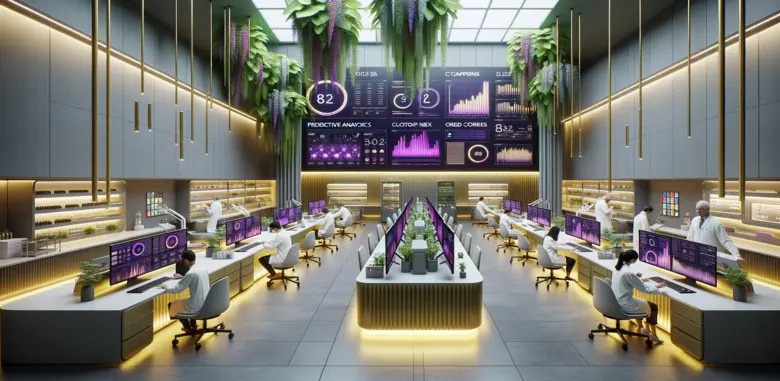
Now let’s see its real-world applications. It will help you better understand healthcare predictive analytics and its working.
Real-world applications of healthcare predictive analytics:
Cancer Detection:
Predictive models analyze a person’s medical history, genetics, and lifestyle to assess their risk of developing cancer. Recommendations for regular screenings, like mammograms or colonoscopies, are tailored to an individual’s risk level. This early detection increases the chances of catching cancer at an early, more treatable stage.
For more information related to this read Machine Learning Applications in Cancer Prognosis and Prediction.
Readmission Prevention:
Predictive analytics assess patient data to identify who is at higher risk of being readmitted to the hospital shortly after being discharged. Patients at higher risk receive extra attention, including follow-up calls, medication reviews, or home visits. By addressing issues promptly, hospitals reduce readmission rates , improve patient outcomes, and save healthcare costs.
Drug Safety:
Pharmaceutical companies use predictive analytics to monitor the safety of new drugs . Algorithms analyze data from clinical trials and real-world patient reports to identify potential adverse reactions. This early detection of safety concerns allows companies to take corrective action, such as revising drug warnings or even withdrawing the medication if necessary, to protect patient safety.
Resource Allocation:
Hospitals use predictive analytics to anticipate spikes in patient admissions during peak seasons like flu outbreaks. By forecasting patient volumes, hospitals can adjust staffing levels and resource allocation accordingly. This ensures that there are enough doctors, nurses, and beds available when they are needed most, improving patient care and reducing wait times.
In these real-world applications, healthcare predictive analytics plays a critical role in improving patient care, safety, and healthcare efficiency. It’s all about using data to make informed decisions that benefit both patients and healthcare providers.
Challenges in Healthcare Predictive Analytics:
Healthcare predictive analytics offers tremendous benefits, but it also faces several challenges:
Data Quality and Integration: Healthcare data, stemming from an array of sources like electronic health records, wearable devices, and social determinants of health, can be a messy affair. Its quality, accuracy, and compatibility are crucial elements to consider. This task can prove quite complicated.
Privacy and Security: With the sensitivity of patient data being a key concern, the need for privacy and security is paramount. Constant worries regarding unauthorized access and data breaches persist, necessitating compliance with standards like HIPAA (Health Insurance Portability and Accountability Act).
Ethical Considerations: Ethical implications are significant when decisions are made using predictive analytics. There’s a risk of unfair treatment or discrimination against certain groups if algorithms aren’t carefully designed, resulting in bias.
Interpreting Complex Models: Some predictive models, like deep learning algorithms, can be complex and challenging to interpret. This makes it difficult for healthcare providers to trust and understand the recommendations, limiting their adoption.
Data Volume and Processing: Healthcare generates massive amounts of data, and processing this data in real time can be computationally intensive. It requires robust infrastructure and substantial computational resources.
Regulatory Compliance: Healthcare is heavily regulated, and implementing predictive analytics solutions must comply with strict healthcare regulations. Navigating these regulations can be complex and time-consuming.
Patient Consent: Obtaining informed consent from patients for data usage and predictive analysis can be challenging. Patients need to understand how their data will be used and for what purpose.
Data Bias: Data used to train predictive models may contain biases that reflect historical disparities in healthcare. These biases can lead to inaccurate predictions and reinforce existing healthcare inequalities.
Physician Adoption: Healthcare providers may be resistant to adopting new technologies, including predictive analytics, due to concerns about job displacement, a lack of trust in algorithms, or a steep learning curve.
Cost and ROI: Implementing predictive analytics systems can be costly, and the return on investment (ROI) may not always be immediately evident. Demonstrating the value of these systems can be a challenge for healthcare organizations.
Long-Term Monitoring: Predictive models require continuous monitoring and updating to remain accurate over time. This necessitates ongoing maintenance and resource allocation.
Addressing these challenges requires a collaborative effort from healthcare organizations, technology developers, policymakers, and researchers to ensure that healthcare predictive analytics can reach its full potential while safeguarding patient privacy, equity, and ethical considerations.
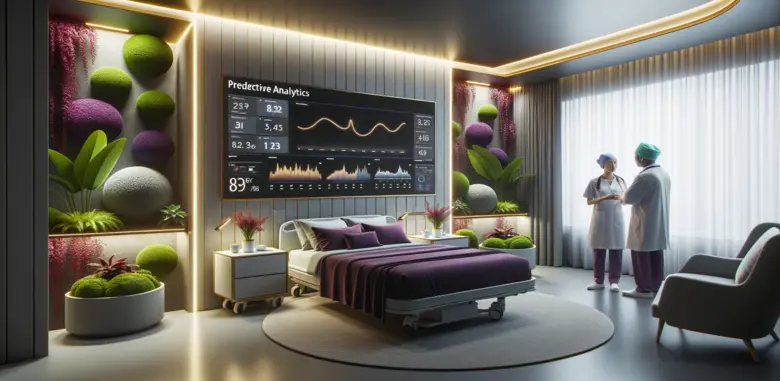
Software Tools of predictive analytics in healthcare:
The software tools and platforms that enable healthcare organizations to utilize the power of predictive analytics, leading to more accurate predictions, improved patient outcomes and better resource allocation while ensuring data security and compliance with healthcare regulations are as follows:
Electronic Health Records (EHRs): EHR systems like Epic, Cerner, and Allscripts store and manage patient data, providing structured clinical information for analysis.
Data Warehousing: Healthcare organizations often use data warehousing solutions like Microsoft SQL Server, Oracle Exadata, and Amazon Redshift to consolidate and store healthcare data.
Machine Learning Algorithms: Various software libraries and tools for machine learning include sci-kit-learn (Python), TensorFlow, and Keras for developing predictive models in healthcare.
Natural Language Processing (NLP): NLP software like Apache OpenNLP, spaCy, and NLTK is employed to extract insights from unstructured clinical notes and text-based data.
Statistical Analysis Software: Statistical packages like R and Python, with libraries such as RStudio and Jupyter Notebooks, are commonly used for building predictive models and conducting statistical analysis.
Predictive Modeling Platforms: Specialized platforms like IBM Watson Health and SAS Healthcare Analytics offer pre-built models and tools designed specifically for healthcare data analytics.
Risk Stratification Tools: Software solutions like Optum360’s Risk Assessment and Stratification Tool (RAST) help categorize patients into risk groups based on predictive analytics.
Geospatial Analytics: Geographic information systems (GIS) software like Esri’s ArcGIS is used for analyzing healthcare data in relation to geographical locations.
Clinical Decision Support Systems (CDSS): CDSS software integrates predictive analytics into clinical workflows. Examples include IBM Watson for Oncology and Epic’s CDS functionality.
Wearable Devices and IoT: Data collected from wearable health devices can be integrated into predictive analytics systems using platforms like Fitbit Health Solutions or Apple HealthKit.
Telemedicine and Remote Monitoring: Telehealth platforms like Teladoc and remote monitoring solutions like Philips’ eCareCompanion provide valuable patient data for predictive analytics.
Predictive Analytics Dashboards: Visualization tools such as Tableau, Power BI, and QlikView allow healthcare professionals to interact with predictive analytics results through customized dashboards.
Cloud Computing: Cloud platforms like Amazon Web Services (AWS), Microsoft Azure, and Google Cloud provide scalable infrastructure for handling large healthcare datasets and analytics processes.
Data Privacy and Security Tools: Tools like Symantec Data Loss Prevention and McAfee offer security measures to protect sensitive healthcare data.
Conclusion:
Healthcare Predictive Analytics is a transformative force in the healthcare industry. As you have read in the blog it enables early warnings, cost savings, smart planning, and personalized care. This benefits patients by improving health outcomes and also enhances the efficiency of healthcare systems is an edge for healthcare industries to work better. Healthcare professionals can identify health risks, intervene timely, and allocate resources effectively, resulting in better patient care and significant cost reduction. Moreover, predictive analytics has real-world applications in areas like cancer detection, readmission prevention, drug safety, and resource allocation, further demonstrating its impact on patient safety and healthcare efficiency.
See the Predictive Analysis implementation in Supply Chain.
This field also faces challenges, including data quality, privacy, ethical considerations, and the need for physician adoption. Addressing these challenges will be essential to realizing the full potential of predictive analytics in healthcare. Predictive analytics will play a central role in shaping the healthcare landscape, ultimately improving patient outcomes and the overall healthcare experience.
Learn how predictive analytics is reshaping marketing strategies in our recent article on Marketing Predictive Analytics.
If you’re interested in how predictive analytics is revolutionizing HR practices, check out our in-depth blog on HR Predictive Analytics.
[h1]https://www.sciencedirect.com/science/article/pii/S2001037014000464
[h2]https://link.springer.com/article/10.1007/s11739-022-03099-2
[h3]https://link.springer.com/chapter/10.1007/978-3-031-20730-3_26
[h4]https://ieeexplore.ieee.org/stamp/stamp.jsp?arnumber=10103533
[h5]https://solutyics.com/marketing-predictive-analytics/
[h6]https://medium.com/@solutyics/hr-predictive-analytics-6f2644eb234f
Author
Team Solutyics is a dynamic group of Analytics and AI specialists who bring together a rich mix of expertise. Their combined insights ensure that readers gain a deeper understanding of practical applications of Analytics and AI.
Comment (1)
[…] sector analytics can be applied across various domains, including healthcare, education, transportation, public safety, social services, and more. It enables government […]