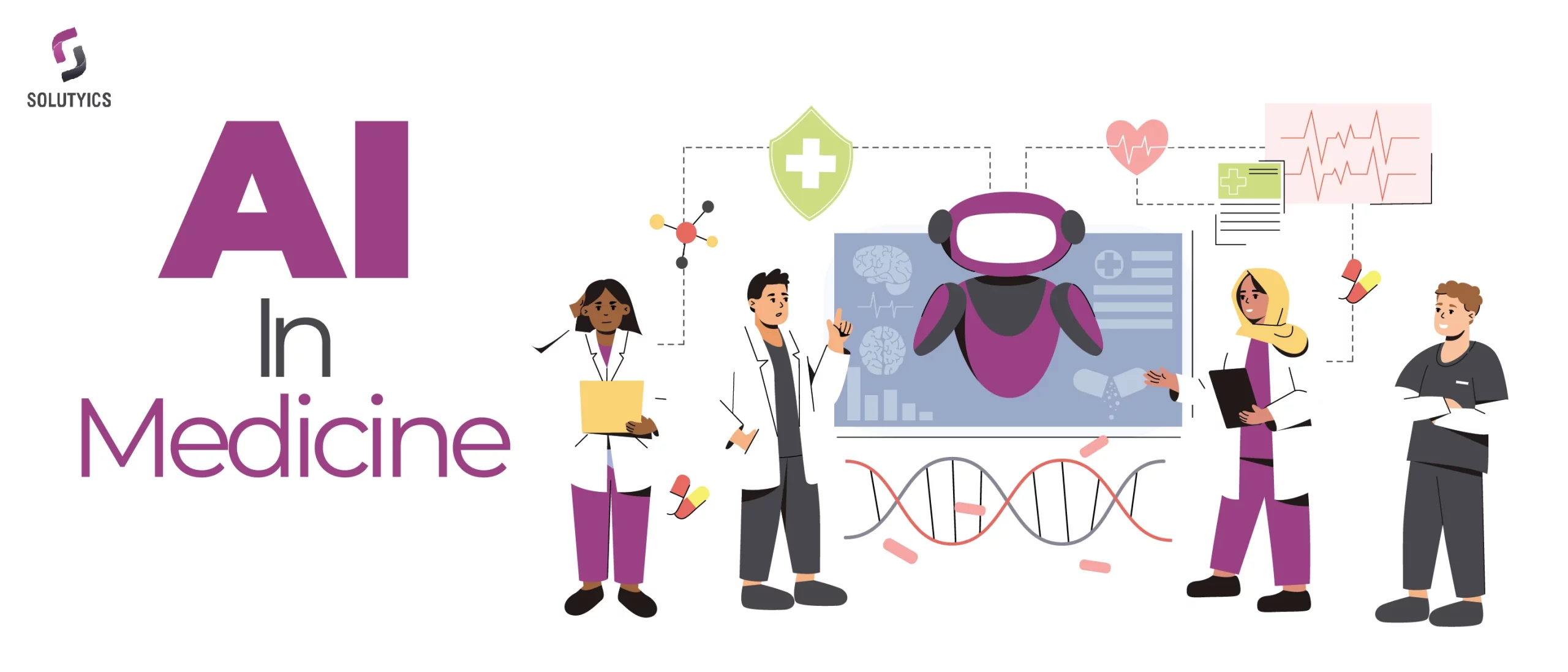
Artificial Intelligence (AI) has emerged as a transformative force in the field of medicine, revolutionizing the way we approach healthcare. AI, a branch of computer science that enables machines to perform tasks that typically require human intelligence, has found profound applications in various medical domains. From diagnostics and treatment planning to patient care and administrative tasks, AI is reshaping the entire healthcare ecosystem.
In the realm of medicine, AI is not just a futuristic concept but a tangible reality that is already making significant strides. This includes the utilization of advanced algorithms, machine learning, and robotics to analyze complex medical data, interpret images, and even assist in surgical procedures. As we stand at the intersection of technology and healthcare, the integration of AI promises to enhance medical capabilities, improve patient outcomes, and usher in a new era of precision and efficiency.
Significance of AI Advancements:
The significance of AI advancements in medicine cannot be overstated. These technological strides bring forth a multitude of benefits that have the potential to transform healthcare on a global scale. Some key aspects of the significance include:
- Precision in Diagnostics: AI algorithms can analyze vast datasets with unparalleled speed and accuracy, aiding healthcare professionals in diagnosing diseases at earlier stages and with greater precision.
- Personalized Treatment Plans: By understanding individual patient data, AI contributes to the development of personalized treatment plans, considering genetic factors, lifestyle, and specific health conditions.
- Efficient Healthcare Processes: Automation of administrative tasks, data analysis, and routine processes allows healthcare providers to focus more on patient care, reducing administrative burdens and optimizing resource allocation.
- Innovative Medical Technologies: AI is driving the development of innovative medical technologies, from robotic-assisted surgeries to virtual health assistants, creating new possibilities for improved patient care and outcomes.
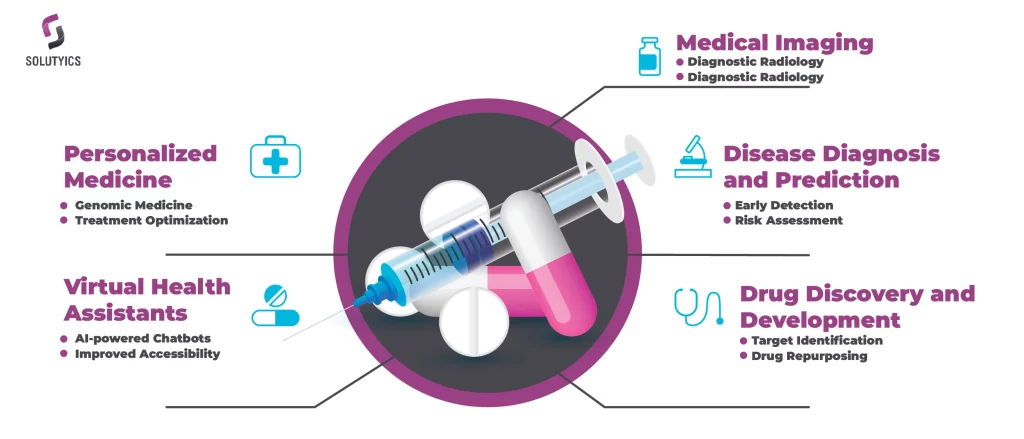
Current Landscape of AI in Medicine
Applications and use cases:
- Medical Imaging:
- Diagnostic Radiology: AI algorithms assist in the interpretation of X-rays, CT scans, and MRIs, aiding radiologists in detecting abnormalities and providing more accurate diagnoses.
- Pathology: AI enhances the analysis of pathology slides, improving the efficiency and accuracy of cancer diagnosis and other diseases.
- Disease Diagnosis and Prediction:
- Early Detection: AI models analyze patient data to identify subtle patterns indicative of early-stage diseases, enabling early intervention and improved prognosis.
- Risk Assessment: Predictive analytics help assess individual patient risks for diseases based on factors such as genetics, lifestyle, and medical history.
- Drug Discovery and Development:
- Target Identification: AI accelerates the identification of potential drug targets by analyzing biological data and predicting the therapeutic relevance of specific molecules.
- Drug Repurposing: AI algorithms analyze existing drugs for new therapeutic uses, potentially speeding up the drug development process.
- Personalized Medicine:
- Genomic Medicine: AI analyzes genomic data to tailor treatments based on an individual’s genetic makeup, optimizing drug efficacy and minimizing side effects.
- Treatment Optimization: Personalized treatment plans are developed through AI analysis, considering patient-specific factors and treatment response data.
- Virtual Health Assistants:
- AI-powered chatbots and virtual assistants provide patients with medical information, answer queries, and offer guidance on managing health conditions, improving accessibility to healthcare resources.
Success Stories and Innovations:
- IBM Watson for Oncology:
- Application: IBM Watson analyzes vast amounts of medical literature, clinical trial data, and patient records to assist oncologists in creating personalized cancer treatment plans.
- Impact: Improved treatment recommendations and access to the latest research contribute to more effective and individualized cancer care.
- Google’s DeepMind in Ophthalmology:
- Application: DeepMind’s AI is employed to analyze eye scans for early signs of diseases like diabetic retinopathy and macular degeneration.
- Impact: Early detection of eye diseases, allowing for timely interventions and preventing vision loss.
- PathAI in Pathology:
- Impact: Increased accuracy and efficiency in pathology diagnoses, leading to better patient outcomes.
- Application: PathAI uses machine learning to assist pathologists in diagnosing diseases from pathology slides.
- Tempus for Clinical and Molecular Data Analysis:
- Application: Tempus utilizes AI to analyze clinical and molecular data to support oncologists in making data-driven decisions.
- Impact: Enhanced treatment strategies, identification of clinical trial opportunities, and continuous learning for improved cancer care.
Natural Language Processing in Healthcare:
- Electronic Health Record (EHR) Analysis:
- Applications: Natural Language Processing (NLP) extracts valuable information from unstructured clinical notes, medical reports, and patient records within EHR systems.
- Benefits: Efficient data extraction, improved interoperability, and a comprehensive understanding of patient histories for better-informed decisions.
- Clinical Documentation and Voice Recognition:
- Applications: NLP-powered tools convert spoken words into structured text, aiding healthcare professionals in documenting patient encounters seamlessly.
- Benefits: Time-saving in documentation, reduced administrative burden, and increased accuracy in capturing patient information.
- Sentiment Analysis and Patient Feedback:
- Applications: NLP analyzes patient feedback from various sources, including surveys and social media, to gauge sentiments and identify areas for improvement in healthcare services.
- Benefits: Enhanced patient experience, improved healthcare service quality, and proactive response to patient concerns.
Robotics and Automation in Medical Procedures:
- Robot-Assisted Surgery:
- Applications: Robots, controlled by surgeons, assist in performing minimally invasive surgeries with precision and dexterity beyond human capabilities.
- Benefits: Increased surgical precision, reduced invasiveness, shorter recovery times, and improved outcomes for patients.
- Telepresence and Remote Surgery:
- Applications: Robotics enable surgeons to perform procedures from remote locations, expanding access to specialized care in areas with limited resources.
- Benefits: Increased accessibility to expert medical care, especially in rural or underserved regions.
- Rehabilitation and Physical Therapy:
- Applications: Robotics aid in rehabilitation exercises and physical therapy, providing targeted and controlled movements for patients recovering from injuries or surgeries.
- Benefits: Customized rehabilitation plans, consistent monitoring, and accelerated recovery processes.
Predictive Analytics for Disease Prevention:
- Identifying High-Risk Populations:
- Applications: Predictive analytics assess patient data to identify individuals at a higher risk of developing specific diseases based on genetic, lifestyle, and environmental factors.
- Benefits: Proactive interventions for high-risk populations, personalized preventive measures, and a shift toward preventive healthcare.
- Forecasting Disease Outbreaks:
- Applications: Predictive modeling analyzes epidemiological data to forecast the spread of infectious diseases, allowing for timely public health interventions.
- Benefits: Early containment strategies, efficient allocation of resources during outbreaks, and improved response to public health crises.
- Monitoring Chronic Conditions:
- Applications: Predictive analytics continuously monitors patient data to predict disease exacerbations in chronic conditions like diabetes, enabling early interventions.
- Benefits: Improved management of chronic diseases, reduced hospitalizations, and enhanced quality of life for patients.
Personalized Medicine and Treatment Plans:
- Genomic Medicine and Targeted Therapies:
- Applications: AI analyzes genomic data to identify genetic variations associated with diseases and inform the development of targeted therapies.
- Benefits: Personalized treatment plans, improved treatment efficacy, and minimized side effects through the customization of therapies.
- Optimizing Medication Regimens:
- Applications: AI assesses patient data, including genetics and responses to medications, to optimize drug selection and dosages for individual patients.
- Benefits: Reduced adverse drug reactions, increased medication efficacy, and improved patient adherence to treatment plans.
- Tailored Behavioral Interventions:
- Applications: AI analyzes patient behaviors and preferences to recommend personalized lifestyle interventions, such as dietary plans and exercise regimens.
- Benefits: Increased patient engagement, more effective behavior change, and improved overall well-being.
Enhancing Patient Care:
Virtual Health Assistants:
- 24/7 Health Information Access:
- Applications: Virtual health assistants powered by AI provide round-the-clock access to health information, offering instant responses to user queries and concerns.
- Benefits: Improved healthcare accessibility, timely information for patients, and reduced burden on healthcare hotlines.
- Medication Reminders and Adherence Support:
- Applications: Virtual assistants assist patients in adhering to medication schedules by providing timely reminders and offering information about prescribed medications.
- Benefits: Increased medication adherence, reduced risk of missed doses, and improved overall health outcomes.
- Symptom Triage and Initial Consultations:
- Applications: Virtual health assistants use AI algorithms to triage symptoms, offering initial consultations and recommendations for further medical attention.
- Benefits: Efficient symptom management, early identification of urgent cases, and streamlined healthcare pathways.
Remote Patient Monitoring:
- Continuous Vital Sign Monitoring:
- Applications: Remote monitoring devices equipped with AI track patients’ vital signs, such as heart rate and blood pressure, providing real-time data to healthcare providers.
- Benefits: Early detection of health anomalies, reduced hospital readmissions, and proactive interventions for deteriorating health.
- Chronic Disease Management:
- Applications: AI-enabled remote monitoring supports the management of chronic conditions by tracking relevant health metrics and providing actionable insights.
- Benefits: Improved quality of life for patients with chronic diseases, better disease management, and reduced healthcare costs.
- Post-Surgical and Post-Treatment Monitoring:
- Applications: Patients recovering from surgeries or treatments are monitored remotely, with AI detecting potential complications and facilitating timely interventions.
- Benefits: Enhanced post-treatment care, reduced hospital stays, and optimized recovery processes.
AI-driven Patient Engagement:
- Personalized Health Education:
- Applications: AI analyzes patient data to provide personalized health education materials, catering to individual health literacy levels and preferences.
- Benefits: Increased patient understanding, improved health literacy, and empowerment for active participation in healthcare decisions.
- Behavioral Health Support:
- Applications: AI-driven platforms offer behavioral health support by identifying patterns in patient behavior and providing interventions for mental health and well-being.
- Benefits: Targeted mental health interventions, improved emotional well-being, and increased accessibility to behavioral health resources.
- Feedback and Follow-ups:
- Applications: AI facilitates automated feedback collection and follow-up interactions, ensuring continuous engagement and addressing patient concerns.
- Benefits: Improved patient satisfaction, increased adherence to treatment plans, and proactive management of patient feedback.
Challenges and Ethical Considerations
Data Privacy and Security:
- Sensitive Health Data Handling:
- Challenge: The integration of AI in healthcare involves the processing of sensitive patient health data, raising concerns about data security and the potential for unauthorized access.
- Ethical Considerations: Implementing robust data encryption, access controls, and compliance with data protection regulations (e.g., HIPAA) to safeguard patient privacy.
- Consent and Patient Control:
- Challenge: AI systems may analyze patient data without explicit consent, challenging the principles of patient autonomy and control over personal health information.
- Ethical Considerations: Establishing transparent consent mechanisms, empowering patients to control the use of their data, and promoting informed decision-making.
- Data Breach Risks:
- Challenge: The interconnected nature of healthcare systems increases the risk of data breaches, potentially exposing sensitive health information to unauthorized parties.
- Ethical Considerations: Prioritizing cybersecurity measures, prompt disclosure of breaches, and implementing measures to mitigate the impact on affected individuals.
Bias in AI Algorithms:
- Bias in Training Data:
- Challenge: AI algorithms trained on biased datasets may perpetuate existing disparities and inequalities in healthcare, leading to biased outcomes.
- Ethical Considerations: Regular audits of training data for biases, transparency in algorithm development, and continuous efforts to mitigate and correct bias in AI models.
- Impact on Underserved Populations:
- Challenge: AI applications may disproportionately benefit certain demographic groups, leaving underserved populations with limited access to the benefits of advanced healthcare technologies.
- Ethical Considerations: Ensuring inclusive representation in training data, addressing biases in algorithmic decision-making, and promoting equitable access to AI-driven healthcare solutions.
- Explainability and Accountability:
- Challenge: The complexity of AI algorithms may result in a lack of transparency and interpretability, making it challenging to understand how decisions are reached.
- Ethical Considerations: Prioritizing explainable AI models, establishing accountability frameworks, and ensuring that healthcare professionals can understand and interpret AI-generated recommendations.
Ensuring Ethical AI Practices in Medicine:
- Informed Consent for AI Use:
- Challenge: Ensuring that patients are adequately informed about the use of AI in their healthcare journey and obtaining informed consent for AI-driven interventions.
- Ethical Considerations: Developing clear and comprehensible communication strategies, educating patients about AI applications, and integrating AI-related information into the informed consent process.
- Professional Autonomy and Collaboration:
- Challenge: Balancing the role of AI as a decision support tool with the autonomy of healthcare professionals, and fostering collaboration between AI systems and human expertise.
- Ethical Considerations: Establishing guidelines for AI-human collaboration, emphasizing the complementary nature of AI, and ensuring that ultimate decision-making rests with healthcare professionals.
- Continuous Monitoring and Accountability:
- Challenge: The dynamic nature of AI systems requires ongoing monitoring for performance, ethical considerations, and accountability in case of system failures.
- Ethical Considerations: Implementing continuous monitoring mechanisms, establishing clear accountability frameworks, and learning from system failures to improve AI practices in medicine.
Integration of AI with Healthcare Professionals
1. Augmented Intelligence in Clinical Decision-making:
Overview:
- Definition: Augmented intelligence refers to the collaboration between AI systems and healthcare professionals to enhance clinical decision-making, combining the strengths of both human expertise and machine capabilities.
- Role of AI: AI assists healthcare professionals by analyzing vast datasets, identifying patterns, and providing evidence-based insights to support diagnostic and treatment decisions.
Applications:
1. Diagnostic Assistance:
AI algorithms analyze medical imaging data, pathology slides, and diagnostic tests to aid in the accurate and timely diagnosis of diseases.
2.Treatment Recommendations:
AI systems assess patient data, medical literature, and treatment guidelines to suggest personalized treatment plans, considering individual variations and best practices.
3.Predictive Analytics:
Machine learning models predict patient outcomes, disease progression, and potential complications, helping healthcare professionals proactively plan interventions.
Benefits:
- Enhanced Accuracy: Augmented intelligence improves the accuracy of diagnostic processes and treatment decisions, reducing errors and enhancing patient outcomes.
- Efficient Workflow: Healthcare professionals can leverage AI for data-intensive tasks, allowing them to focus on complex decision-making and patient interactions.
- Continuous Learning: AI systems continuously learn from real-world healthcare scenarios, adapting and improving their recommendations over time.
2. Collaboration between AI Systems and Healthcare Providers:
Overview:
- Definition: Effective collaboration involves integrating AI systems seamlessly into healthcare workflows, ensuring clear communication, and fostering mutual trust between AI technologies and healthcare providers.
- Interdisciplinary Approach: Collaboration extends beyond individual practitioners to involve interdisciplinary teams, including data scientists, clinicians, and IT specialists.
Applications:
1.Clinical Decision Support Systems:
AI systems provide real-time support by offering relevant clinical information, treatment options, and potential risks, aiding healthcare providers in decision-making.
2.EHR Integration:
AI technologies seamlessly integrate with Electronic Health Records (EHRs), enhancing the accessibility and usability of patient data for healthcare professionals.
3.Training and Education:
AI-powered simulations and educational tools assist in the training of healthcare professionals, allowing them to familiarize themselves with novel procedures and technologies.
Benefits:
- Efficient Information Retrieval: AI facilitates quick access to relevant patient information, reducing the time spent on administrative tasks and allowing healthcare providers to focus on patient care.
- Interdisciplinary Collaboration: Integrating AI involves collaboration between healthcare providers and experts in data science, fostering a culture of interdisciplinary teamwork.
- Improved Patient Outcomes: The synergy between AI and healthcare professionals leads to improved diagnostic accuracy, personalized treatment plans, and better overall patient care.
The integration of AI with healthcare professionals represents a transformative paradigm in medicine, where technology acts as a supportive ally, augmenting the capabilities of healthcare providers. As we delve deeper into the impact of this integration, we’ll explore real-world examples and case studies that illustrate the practical applications and benefits of augmented intelligence in clinical settings.
Impact on Healthcare Infrastructure
1. AI and Healthcare Accessibility:
Overview:
Accessibility Enhancement: The integration of AI in healthcare contributes to improved accessibility by overcoming geographical, financial, and resource-related barriers, thereby enhancing healthcare delivery.
Applications:
1.Telemedicine and Remote Consultations:
AI supports telemedicine initiatives by providing remote diagnostic assistance, facilitating virtual consultations, and enabling healthcare access in underserved areas.
2.Chatbots and Virtual Health Assistants:
AI-powered chatbots and virtual health assistants offer immediate health information and guidance, addressing basic queries and concerns without the need for direct human interaction.
3.Predictive Analytics for Resource Allocation:
AI helps predict healthcare service demands, enabling proactive resource allocation and ensuring that medical services are available where and when they are needed most.
Benefits:
- Increased Reach: AI-driven telemedicine and virtual health assistants extend healthcare services to remote and underserved populations, increasing overall healthcare accessibility.
- Timely Interventions: Predictive analytics assist in identifying areas with potential healthcare service gaps, allowing for timely interventions and resource allocation.
- Reduced Healthcare Disparities: AI interventions contribute to minimizing healthcare disparities by providing equitable access to information and services.
2. Economic Implications and Resource Optimization:
Overview:
Efficiency and Cost-effectiveness: The integration of AI in healthcare has economic implications, optimizing resource utilization, reducing operational costs, and improving overall healthcare efficiency.
Applications:
1.Operational Efficiency in Hospitals:
AI streamlines hospital workflows, optimizing administrative processes, reducing waiting times, and enhancing overall operational efficiency.
2.Resource Allocation and Planning:
Predictive analytics assist healthcare institutions in optimizing resource allocation, preventing overutilization or underutilization of facilities, staff, and equipment.
3.Cost-effective Diagnostics:
AI-powered diagnostic tools and imaging analysis contribute to cost-effective and timely diagnostics, reducing the need for extensive and expensive diagnostic procedures.
Benefits:
- Cost Savings: AI-driven efficiencies result in cost savings for healthcare institutions, potentially leading to reduced healthcare costs for patients.
- Optimized Resource Utilization: Predictive analytics help prevent resource shortages or surpluses, ensuring that healthcare resources are allocated where they are most needed.
- Improved Financial Sustainability: Economic implications of AI contribute to the financial sustainability of healthcare systems, potentially making quality healthcare more accessible to a larger population.
Case Studies: Real-world Examples of Successful AI Implementations in Medicine
1. IBM Watson for Oncology:
- Overview:
Application: IBM Watson for Oncology is an AI-powered platform designed to assist oncologists in making evidence-based treatment decisions for cancer patients.
Functionality: The platform analyzes a vast amount of medical literature, clinical trial data, and patient records to provide personalized treatment recommendations.
- Success Factors:
Enhanced Treatment Planning: Watson for Oncology supports oncologists in identifying optimal treatment options based on the latest medical knowledge and patient-specific factors.
Global Impact: Deployed in multiple countries, the platform has demonstrated its potential to impact cancer care on a global scale.
2. Google’s DeepMind in Ophthalmology:
- Overview:
Application: DeepMind, a subsidiary of Google, has developed AI algorithms for the analysis of optical coherence tomography (OCT) scans in ophthalmology.
Functionality: The AI system can detect and diagnose eye conditions, including age-related macular degeneration and diabetic retinopathy, from OCT scans.
- Success Factors:
Early Disease Detection: DeepMind’s AI demonstrates the ability to detect signs of eye diseases at an early stage, enabling timely intervention and treatment.
Integration into Clinical Workflow: The system is designed to seamlessly integrate into the existing clinical workflow, assisting ophthalmologists in their diagnostic processes.
3. PathAI in Pathology:
- Overview:
Application: PathAI leverages AI to assist pathologists in diagnosing diseases from pathology slides, particularly in the field of pathology and histopathology.
Functionality: The platform aids in the identification and classification of diseases, improving diagnostic accuracy.
- Success Factors:
Precision in Diagnosis: PathAI enhances the precision of pathology diagnoses by providing additional insights and reducing the likelihood of human error.
Collaboration with Pathologists: The system operates as a supportive tool for pathologists, fostering collaboration between AI technology and human expertise.
4. Tempus for Clinical and Molecular Data Integration:
- Overview:
Application: Tempus integrates clinical and molecular data using AI to empower healthcare professionals with comprehensive insights for cancer care.
Functionality: The platform analyzes diverse data sources, including clinical records and molecular data, to guide personalized treatment plans.
- Success Factors:
Personalized Treatment Plans: Tempus enables the generation of personalized treatment plans by considering both clinical and molecular characteristics of cancer patients.
Data-driven Decision Support: Healthcare professionals benefit from AI-driven decision support, facilitating data-driven discussions about optimal treatment strategies.
Regulatory Landscape
1. Current Regulations for AI in Medicine:
Overview:
- FDA Oversight: In the United States, the Food and Drug Administration (FDA) plays a key role in regulating AI applications in medicine, particularly those considered as medical devices.
- Risk-Based Approach: Regulations focus on ensuring the safety and effectiveness of AI technologies, with a risk-based approach that considers the potential impact on patient health.
Key Considerations:
- FDA Approval for Medical Devices: AI applications intended for medical diagnosis, treatment recommendations, or decision support are subject to FDA approval, similar to traditional medical devices.
- Quality System Regulation (QSR): Manufacturers of AI-based medical devices must comply with QSR requirements to ensure the quality and reliability of their products.
- Software as a Medical Device (SaMD): AI software that meets the definition of SaMD is subject to regulatory requirements, emphasizing factors such as clinical evaluation and validation.
2. Anticipated Changes and Challenges:
Overview:
- Evolution of Technology: The rapid evolution of AI technologies poses challenges for regulatory frameworks to keep pace with innovations while maintaining patient safety.
- Interdisciplinary Collaboration: Addressing the complexities of AI in medicine requires collaboration between regulatory bodies, healthcare professionals, and technology developers.
Anticipated Changes:
- Adaptive Regulatory Approaches: Regulators are exploring adaptive approaches to accommodate the dynamic nature of AI, considering the continuous learning and updating of algorithms.
- International Harmonization: Efforts are underway to harmonize international regulations for AI in medicine, facilitating consistency and efficiency in approvals across borders.
- Real-world Evidence (RWE): Increasing emphasis on leveraging real-world evidence in the regulatory process to complement traditional clinical trial data and facilitate faster approvals.
Challenges:
- Algorithm Transparency: Ensuring transparency in AI algorithms, especially in complex deep learning models, remains a challenge for regulators in understanding and validating decision-making processes.
- Ethical Considerations: The regulatory landscape is grappling with ethical considerations, including biases in AI algorithms, patient consent, and the equitable distribution of benefits.
- International Variability: Divergence in regulatory approaches across countries poses challenges for global implementation, requiring coordination to avoid barriers to innovation.
As AI continues to play an increasingly prominent role in medicine, the regulatory landscape is evolving to balance innovation with patient safety and ethical considerations. Anticipated changes aim to foster a regulatory environment that promotes the responsible development and deployment of AI technologies in healthcare.
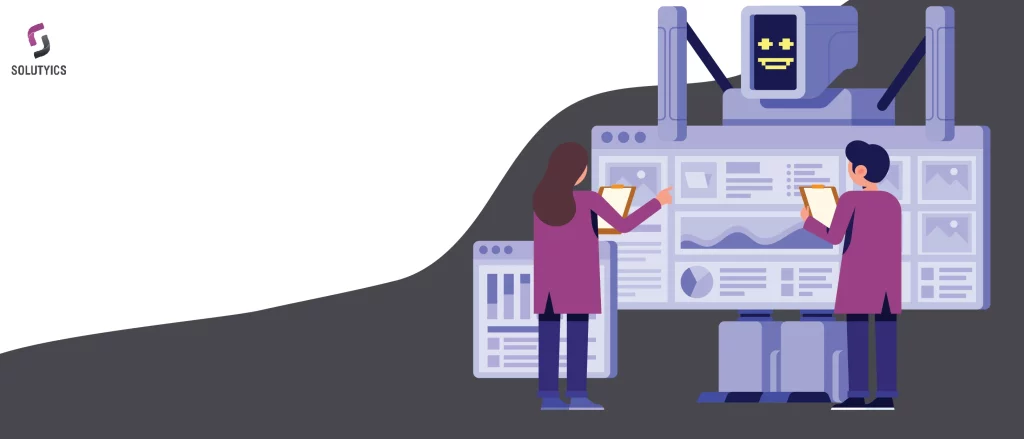
Future Trends and Possibilities
1. Emerging Technologies in AI for Medicine:
Overview:
- Integration of Multi-Modal Data: Future AI applications in medicine will likely involve the integration of diverse data types, including genomics, imaging, clinical records, and real-time patient monitoring.
- Explainable AI (XAI): Advancements in Explainable AI aim to enhance the interpretability of AI models, making their decision-making processes more understandable and transparent.
- Edge AI in Medical Devices: The use of Edge AI, processing data closer to the source, is expected to become more prevalent, especially in medical devices and wearables.
Implications:
- Holistic Patient Insights: Multi-modal data integration enables a more comprehensive understanding of individual patient profiles, leading to personalized and targeted interventions.
- Trust and Adoption: Explainable AI addresses concerns related to trust and acceptance by providing clear explanations for AI-driven decisions, fostering better collaboration between AI systems and healthcare professionals.
- Real-time Decision-making: Edge AI in medical devices allows for real-time processing of patient data, enabling timely interventions and reducing dependence on centralized systems.
2. Predictions for the Next Decade:
Overview:
- AI-driven Drug Discovery: AI is anticipated to play a pivotal role in accelerating drug discovery processes by predicting potential drug candidates, optimizing clinical trial designs, and identifying personalized treatment approaches.
- Enhanced Diagnostics with Biomarkers: AI applications are expected to leverage biomarkers and molecular diagnostics for more accurate and early disease detection, improving diagnostic precision.
- Personalized Mental Health Interventions: AI is predicted to contribute to personalized mental health interventions by analyzing behavioral patterns, predicting mental health risks, and offering tailored therapeutic strategies.
Implications:
- Accelerated Drug Development: AI-driven predictions and simulations expedite the drug discovery and development pipeline, potentially leading to faster availability of novel therapeutics.
- Precision Medicine Advancements: The integration of AI with biomarkers enhances the precision of diagnostic processes, allowing for more targeted and effective treatment strategies.
- Data-driven Mental Health Support: AI applications in mental health provide personalized insights and interventions, contributing to more effective and individualized mental healthcare.
The future of AI in medicine holds exciting possibilities, driven by emerging technologies and a deepening understanding of the potential applications of artificial intelligence. As we look ahead to the next decade, these trends and predictions signify a transformative era in healthcare, where AI plays a central role in advancing medical research, improving patient outcomes, and reshaping the landscape of healthcare delivery.
Conclusion:
In conclusion, the integration of Artificial Intelligence (AI) in medicine marks a transformative leap toward a more efficient, personalized, and accessible healthcare landscape. The advancements in machine learning, natural language processing, and robotics are reshaping diagnostics, treatment plans, and patient care. Success stories illustrate the tangible impact of AI on medical imaging, disease prevention, and clinical decision-making.
However, this paradigm shift comes with challenges. Issues such as data privacy, algorithmic bias, and ethical considerations demand careful attention. Striking a balance between innovation and ethical practices, ensuring collaboration between AI systems and healthcare professionals, and addressing accessibility concerns are pivotal for the sustained success of AI in medicine.
Looking forward, the future promises even more groundbreaking technologies and applications. The regulatory landscape will evolve to keep pace with technological advancements, presenting both opportunities and challenges. As we envision the next decade, AI holds the potential to revolutionize healthcare further, optimizing resources, enhancing economic implications, and ultimately improving patient outcomes.
The journey of AI in medicine is an ongoing story of innovation, challenges, and potential. It is a journey that requires collective efforts from healthcare professionals, technologists, and policymakers to harness the full benefits of AI while upholding the highest standards of ethics and accessibility. The future healthcare landscape is poised for remarkable transformation, driven by the power of Artificial Intelligence.
Author
Team Solutyics is a dynamic group of Analytics and AI specialists who bring together a rich mix of expertise. Their combined insights ensure that readers gain a deeper understanding of practical applications of Analytics and AI.
Comments (3)
I am not typically one to read blog posts; however, this article almost compelled me to do so. Your writing style has truly impressed me. Many thanks for this excellent post.
I luckily stumbled upon this wonderful website a few days back, they offer helpful content for members. The site owner is doing a terrific job serving the community. I’m thrilled and hope they persist in their excellent service.
This post is so informative. I’m glad I read it.