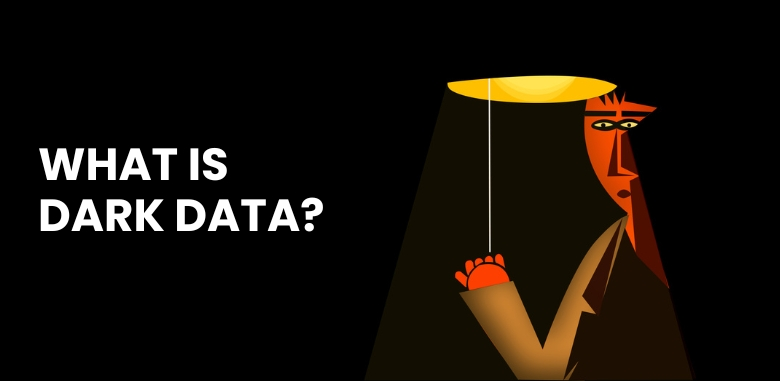
Dark data, a term gaining prominence in the data landscape, refers to the vast amount of data collected during routine business operations that remains unanalyzed or underutilized. This untapped data encompasses various forms, including text, images, documents, and other unstructured formats. Unlike structured data that is organized and actively used, dark data stays in the shadows, holding potential insights and valuable information that organizations often overlook.
Understanding the Concept of Dark Data
Dark data is generated from diverse sources such as customer interactions, sensor outputs, logs, and legacy systems. Despite its potential, it often resides in storage without being processed or analyzed. Uncovering the value within dark data requires recognizing that each piece of information, no matter how seemingly insignificant, holds the possibility of revealing patterns, trends, and connections that can drive informed decision-making and innovation.
Types of Dark Data
Dark data takes on various forms, each offering distinct insights when brought to light. Let’s explore these types:
Unstructured Dark Data
Unstructured dark data refers to data that lacks a predefined or organized format, making it difficult to analyze using traditional methods. This data type includes text, images, audio files, video content, and other information that doesn’t fit neatly into structured databases or spreadsheets. Examples of unstructured dark data include customer reviews, social media posts, emails, and multimedia content.
Because of its lack of structure, unstructured dark data can be challenging to process and interpret. However, advancements in natural language processing and machine learning have made it possible to extract valuable insights from unstructured data sources.
Semi-Structured Dark Data
Semi-structured dark data refers to data that has some level of organization but doesn’t stick to a strict, predefined structure like traditional databases. It falls between fully structured and unstructured data categories. This type of data often includes metadata or tags that provide some context, making it more accessible than completely unstructured data.
So, in simple terms, semi-structured dark data is a bit like a mix of organized and messy information with some helpful notes to make sense of it. It’s not as tidy as traditional databases but not as chaotic as completely unorganized data. Examples of semi-structured dark data include XML files, JSON files, emails, and documents with embedded data. While these formats might have elements of organization, they still lack the rigid structure of data stored in relational databases. Analyzing semi-structured dark data requires techniques that can handle its varying formats and levels of organization.
Structured Dark Data: Hidden Treasures
Structured dark data refers to data that is organized and stored in a structured format, similar to traditional databases. However, despite its organized nature, this data remains unanalyzed or underutilized. Unlike unstructured or semi-structured data, structured dark data is stored in well-defined tables, rows, and columns, making it readily accessible for analysis.
Examples of structured dark data include customer transaction records, sales data, inventory lists, and financial statements. This data type is often generated as part of regular business operations but might not be actively analyzed to derive insights.
Analyzing structured dark data can provide organizations with valuable business intelligence, helping them identify trends, patterns, and opportunities for optimization. By unlocking the potential within structured dark data, organizations can make informed decisions and drive strategic growth.
Understanding these dark data types empowers organizations, unlocking doors to uncharted insights, enhancing decision-making, and propelling innovation forward. The diverse sources and forms of dark data provide a wealth of possibilities waiting to be harnessed.
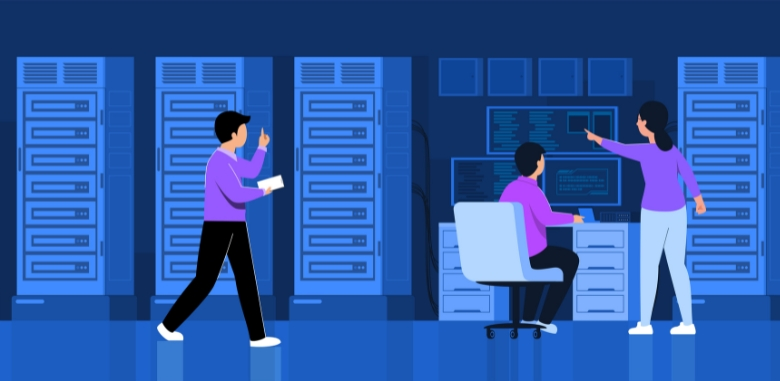
Significance of Dark Data Analytics
The significance of dark data analytics lies in its potential to transform overlooked, untapped information into valuable insights and strategic advantages. By analyzing dark data, organizations can:
- Enhance Decision-Making: Dark data often contains hidden patterns and trends that can inform better decision-making across various business functions.
- Drive Innovation: Unexplored data sources can yield innovative ideas and opportunities that traditional analysis might overlook.
- Improve Customer Understanding: Analyzing customer interactions from dark data can provide deeper insights into preferences and behaviors.
- Optimize Operations: Extracting insights from dark data can lead to process improvements and operational efficiencies.
- Mitigate Risks: By uncovering hidden risks and vulnerabilities, organizations can proactively address issues before they escalate.
- Achieve Competitive Edge: Leveraging insights from dark data can lead to unique strategies that differentiate an organization from competitors.
- Enhance Data Monetization: Monetizing dark data can open new revenue streams and business models.
- Reduce Carbon Footprint: Proper analysis and utilization of dark data can help organizations minimize wasteful data storage practices.
In essence, dark data analytics transforms unutilized information into actionable intelligence, propelling organizations toward informed decisions, innovation, and improved overall performance.
Dark Data Examples:
- CCTV Footage: Surveillance cameras in retail stores capture hours of video footage daily, but much of it goes unexamined. Analyzing this footage can reveal customer behavior patterns, store layout optimization, and potential security risks. Retail stores use closed-circuit television (CCTV) cameras to monitor their premises and ensure security. However, the sheer volume of video data generated often exceeds the capacity of human observers to analyze every minute detail. This unanalyzed video data becomes a prime example of dark data. Analyzing this dark data—CCTV footage—can unlock a wealth of insights. For instance, by examining customer movement and dwell times, retailers can discern popular store sections and products. This information informs store layout optimization, enabling strategic placement of high-demand items for increased exposure and sales. Furthermore, dark data analytics can help identify customer behavior patterns. By tracking the routes customers take through the store, retailers gain a better understanding of shopping habits, allowing them to tailor marketing strategies and product placements accordingly. Security is another critical aspect. Analyzing CCTV footage can help detect unusual or suspicious behavior, potentially preventing theft, damage, or other security breaches. This proactive approach enhances store security and minimizes losses. In essence, transforming unanalyzed CCTV footage from dark data into actionable insights empowers retailers to make informed decisions about store layout, marketing strategies, and security measures, ultimately contributing to improved customer experiences and business outcomes.
- Smart Home Device Data: In modern homes equipped with smart devices like thermostats, lights, and appliances, data is generated as residents interact with these technologies. This data, which includes usage patterns, temperature settings, and device activations, often goes unnoticed. Analyzing this dark data can unveil valuable insights into residents’ routines, preferences, and energy consumption habits. For instance, recognizing when certain devices are used most frequently or identifying trends in temperature adjustments can guide energy-efficient practices. Additionally, understanding user behaviors with smart devices can inform product improvements and customization options, enhancing the overall smart home experience while conserving resources.
- Library Check-Out Records: Public libraries collect extensive data on the books and materials that patrons borrow. However, this data often remains untouched after the initial transaction. Analyzing this dark data can provide valuable insights into reading preferences, popular genres, and emerging trends within the community. By identifying frequently borrowed materials and patterns of interest, libraries can curate their collections more effectively, ensuring they offer materials that resonate with their patrons. This data can also be used to organize relevant events, workshops, or book clubs, fostering community engagement and promoting literacy. Transforming these check-out records from dark data to actionable insights enriches the library’s offerings and strengthens its connection with its users.
- Public Transportation Ticketing Data: Urban transit systems gather vast amounts of data through ticketing systems, tracking routes, timings, and passenger volumes. Yet, this data often remains untapped. Analyzing this dark data can yield valuable insights into commuting patterns, peak travel times, and popular routes. By understanding how people move within a city, transportation authorities can optimize schedules, allocate resources efficiently, and plan infrastructure improvements. This analysis can lead to reduced congestion, improved service quality, and enhanced overall transportation experiences for residents and visitors alike. By shedding light on this hidden data, urban planners can make informed decisions that positively impact public transit systems and urban mobility.
Challenges in Dealing with Dark Data
Dealing with dark data comes with its own set of challenges, which organizations need to navigate to unlock its potential:
- Data Discovery: Identifying and locating dark data within an organization’s vast repositories can be complex, as this data often resides in various formats and storage locations.
- Data Quality: Dark data may lack standardization and could be incomplete, leading to inaccurate or unreliable insights when analyzed.
- Data Security: Analyzing dark data requires careful handling to ensure sensitive information is protected and compliant with data privacy regulations.
- Data Integration: Integrating and harmonizing dark data with existing datasets can be challenging due to varying formats and structures.
- Resource Allocation: Analyzing dark data demands computational resources, from storage space to processing power, which may require investment and optimization.
- Data Governance: Establishing governance policies for handling and analyzing dark data is crucial to ensure ethical and legal compliance.
- Lack of Expertise: Organizations may lack the necessary skills and expertise to effectively analyze unstructured or complex dark data.
- Cost: Extracting insights from dark data requires investment in analytics tools, infrastructure, and skilled personnel.
- Time-Consuming Analysis: Analyzing unstructured data can be time-consuming, as it involves preprocessing and transforming data into a usable format.
- Changing Landscape: Dark data challenges evolve as technology advances and new sources of data emerge, requiring organizations to stay adaptable.
- Privacy Concerns: Analyzing certain types of dark data, such as customer interactions, may raise privacy concerns, requiring organizations to balance analysis with data protection.
Navigating these challenges requires a well-defined strategy, the right tools, skilled personnel, and a commitment to data ethics and compliance. Overcoming these obstacles can lead to uncovering valuable insights from previously untapped data sources.
How can Small Businesses use Dark Data?
Dark data analytics strategies involve systematic approaches to extracting insights from unexplored and underutilized data sources. Here are some key strategies:
- Data Discovery: Identify catalog sources of dark data within the organization, including email archives, log files, sensor data, and more.
- Data Classification: Categorize dark data based on relevance, potential value, and regulatory considerations. Prioritize data for analysis.
- Data Cleaning and Preparation: Cleanse and preprocess data to ensure accuracy, consistency, and compatibility with analytics tools.
- Data Integration: Integrate dark data with existing datasets to gain a comprehensive view. This might involve merging structured and unstructured data.
- Advanced Analytics Techniques: Utilize machine learning, natural language processing, and other advanced techniques to uncover patterns and insights within unstructured data.
- Technology Adoption: Implement analytics platforms, tools, and solutions capable of handling unstructured data processing and analysis.
- Skill Development: Train data analysts in techniques specific to handling dark data, as it often requires unique skills and expertise.
- Data Governance: Establish guidelines for analyzing dark data, including data privacy, security, and compliance considerations.
- Use Case Identification: Define specific business use cases for dark data analysis, aligning with strategic objectives and areas for improvement.
- Experimentation and Exploration: Encourage a culture of exploration by allowing analysts to experiment with different approaches and tools to uncover insights.
- Iterative Process: Dark data analytics is an ongoing process. Continuously refine strategies based on lessons learned and emerging technologies.
- Cross-functional collaboration: Engage teams from different departments to ensure insights from dark data are effectively used to drive decision-making.
- Ethical Considerations: Address ethical concerns related to privacy and data usage, ensuring transparency and compliance with regulations.
- Measurement and Evaluation: Define key performance indicators (KPIs) to measure the impact of dark data analytics on business outcomes.
By employing these strategies, organizations can methodically approach dark data analysis, extracting meaningful insights and converting untapped information into a valuable asset for informed decision-making and innovation.
If you are looking detail information about predictive analysis in HR field read the article.
Real-World Applications of Dark data in industries:
Here are real-world applications of dark data analytics in healthcare, finance, and manufacturing:
Healthcare:
- Medical Imaging Analysis: Dark data from medical images like X-rays, MRIs, and CT scans can be analyzed to detect subtle anomalies and patterns that may aid in early disease diagnosis.
- Clinical Trial Optimization: Analyzing historical trial data that often remains unutilized can help researchers identify factors that impact trial success, patient recruitment, and treatment efficacy.
- Pharmacovigilance: Extracting insights from unstructured adverse event reports and patient narratives can enhance drug safety surveillance and regulatory compliance.
- Patient Monitoring: Dark data from wearable devices and health apps can provide continuous patient data for monitoring chronic conditions and predicting health deterioration.
- Finance:
- Fraud Detection: Analyzing unprocessed transaction data can reveal unusual patterns that indicate fraudulent activities, helping financial institutions prevent and mitigate fraud.
- Credit Risk Assessment: Utilizing unstructured data from sources like social media can provide additional information for assessing credit risk of individuals and businesses.
- Market Sentiment Analysis: Analyzing unexplored social media and news data can provide insights into market sentiment, helping traders and investors make informed decisions.
- Customer Behavior Analysis: Extracting insights from unstructured customer interactions and feedback can guide product development, marketing strategies, and customer service improvements.
Manufacturing:
- Predictive Maintenance: Analyzing unprocessed sensor data from manufacturing equipment can predict maintenance needs, reducing downtime and optimizing production processes.
- Supply Chain Optimization: Dark data from logistics and supplier interactions can help optimize inventory management, lead times, and supply chain efficiency.
- Quality Control: Uncovering insights from unexplored data collected during the production process can improve product quality by identifying defects and deviations.
- Energy Consumption Analysis: Analyzing unstructured data from energy meters and utility bills can help manufacturers optimize energy consumption and reduce operational costs.
These examples showcase how dark data analytics can offer tangible benefits across diverse industries by turning overlooked data into actionable insights, ultimately driving improvements in processes, decision-making, and business outcomes.
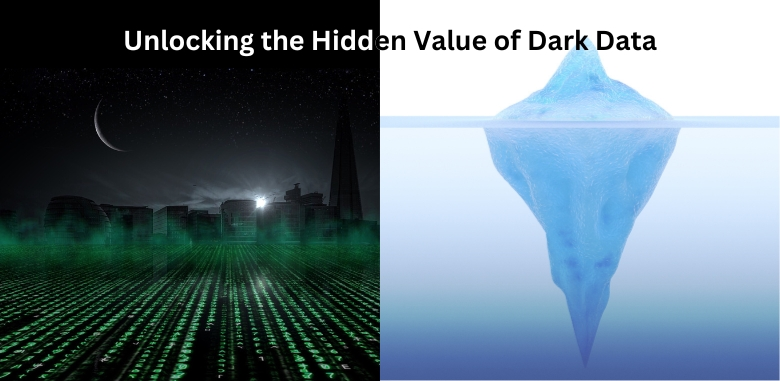
Future Trends in Dark Data Analytics:
Future trends in dark data analytics are poised to shape how organizations utilize untapped data sources for strategic advantage. Some key trends include:
- Automated Data Discovery: Advanced AI-driven tools will facilitate the automated identification and cataloging of dark data sources, streamlining the data discovery process.
- Enhanced Data Quality Solutions: Innovations in data quality tools will address challenges associated with cleansing and preparing unstructured data, ensuring accuracy for analysis.
- AI-Powered Natural Language Processing: AI-driven NLP models will enable more sophisticated analysis of unstructured text data, extracting deeper insights and sentiment analysis.
- Blockchain for Data Security: Blockchain technology will play a role in securing and verifying the authenticity of unprocessed data, fostering trust in the analytics process.
- Edge Analytics: Analytics capabilities will move closer to data sources, enabling real-time analysis of unprocessed data at the edge of networks, and improving responsiveness.
- Federated Learning: Organizations will collaborate to analyze dark data without sharing sensitive data directly, preserving privacy while deriving collective insights.
- Ethics and Governance Solutions: Specialized tools will emerge to ensure the ethical use of dark data, addressing privacy concerns and regulatory compliance.
- Interdisciplinary Collaboration: Teams with diverse expertise, including data scientists, domain specialists, and ethicists, will collaborate to harness insights from dark data effectively.
- Quantum Computing: Quantum computing advancements will enable faster and more complex analysis of unstructured data, unlocking deeper insights.
- Data Monetization Platforms: Platforms facilitating the ethical exchange of dark data insights between organizations will emerge, opening new avenues for revenue generation.
- Hybrid Cloud Solutions: Organizations will use hybrid cloud environments to combine the benefits of cloud-scale analytics with on-premises data control.
- Explainable AI: Ensuring transparency in analytics models will be crucial, particularly when extracting insights from unstructured data, and building trust in decision-making.
As these trends unfold, organizations will be better equipped to harness the potential of dark data, turning unexplored information into actionable insights that drive innovation, efficiency, and competitive advantage.
Conclusion:
Dark data, often overlooked and underutilized, is a hidden asset that holds immense value. It holds the potential to reveal insights that can drive better decisions, innovation, and efficiency across industries. By embracing the challenge of analyzing this unexplored data, organizations can unlock a new level of competitiveness and growth. The journey might be complex, but the rewards—enhanced customer experiences, streamlined operations, and fresh opportunities—are worth the effort. As we navigate this uncharted territory, we step into a future where dark data isn’t just a challenge; it’s a valuable resource that has the power to transform the way we understand and utilize information.
Author
Team Solutyics is a dynamic group of Analytics and AI specialists who bring together a rich mix of expertise. Their combined insights ensure that readers gain a deeper understanding of practical applications of Analytics and AI.