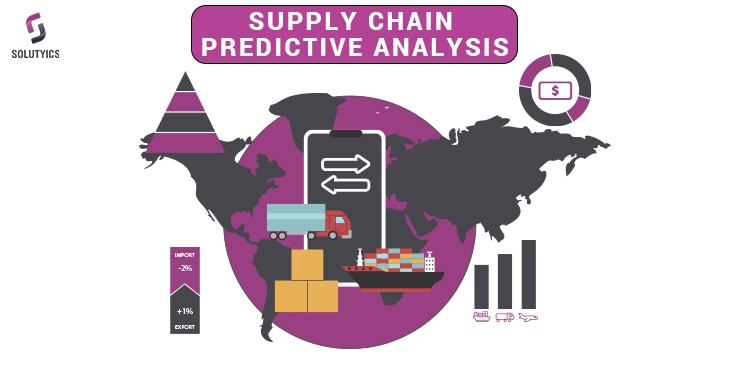
Predictive analytics in the supply chain refers to the use of data, statistical algorithms, and machine learning techniques to forecast future events and trends within the supply chain. It analyzes historical data and then predicts inventory levels, demand, supplier performance, transportation logistics, and other critical factors. By applying predictive analytics in the supply chain, organizations can optimize their supply chain operations, reduce costs, improve efficiency, and enhance overall decision-making, ultimately leading to a more agile and responsive supply chain.
How it works?
Here you might be wondering how It works.
Predictive analytics in the supply chain works by collecting and analyzing large amounts of historical data from various sources such as sales, inventory levels, weather patterns, and supplier performance. Then to identify patterns, correlations, and trends, machine learning algorithms are applied to this data. These algorithms can predict future demand, potential disruptions, and optimal inventory levels.
Steps to apply predictive analytics in the supply chain:
It operates through the following steps:
First of all, by gathering historical data from sources like sales records, inventory levels, customer orders, and external factors (e.g., weather, economic indicators).
Secondly cleaning and preprocessing the data to remove errors, duplicates, and inconsistencies, to make it useful and suitable for analysis.
The third step is choosing appropriate machine learning algorithms, such as regression, time series analysis, or decision trees, based on the specific supply chain problem at hand.
Then use historical data to train the selected algorithms, allowing them to learn patterns and relationships within the data.
After doing the above 4 steps it finally gets capable of predicting. Thinking how? Well by applying the trained model to new data it makes predictions about future events, such as future demand, potential disruptions, or inventory needs.
Now you will start getting rewards for your efforts. These predictions can be used to optimize supply chain decisions such as managing inventory, scheduling production, and distribution planning.
But Remember! You should continuously update and refine the predictive models with new data to improve accuracy and adapt to changing conditions in the supply chain.
By following these steps, organizations can finally utilize the power of predictive analytics to make more informed and proactive decisions in their supply chain operations.
You have read the above steps. We provided you with an overview of supply chain predictive analytics and steps to apply it in the supply chain. You might need more reasons to do so because we only take such actions when we are sure it will give us some reward. Time to tell you those rewards to your business.
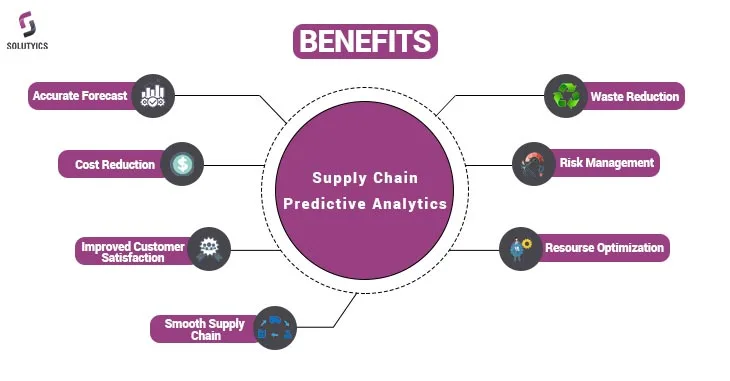
Applying predictive analytics in your supply chain can indeed increase your profits and provide several compelling reasons to do so:
Demand Forecasting Accuracy:
Predictive analytics can improve demand forecasting accuracy. It can understand future customer demand more precisely, helping you reduce excess inventory costs while ensuring you have the right products available when customers want them, ultimately increasing sales and profitability.
For instance, using predictive analytics means you can better predict when people will buy your bread. For instance, if you notice that more bread is sold on sunny weekends, you can make more bread for those days and less on weekdays. This way, you don’t run out of bread on weekends and reduce waste during the week, saving costs and increasing your bakery’s profits.
Cost Reduction:
It can help optimize inventory levels, reduce transportation costs, and minimize production downtime. You can lower operating expenses and increase overall profitability.
For example, a delivery company can use predictive analytics to analyze historical delivery data, traffic patterns, and weather conditions. By doing so, they can optimize delivery routes, ensuring that drivers take the most efficient paths to reach multiple destinations, reducing fuel consumption and transportation time. Additionally, accurate demand forecasting helps them consolidate deliveries and avoid unnecessary trips, further cutting transportation costs while increasing overall profitability.
Improved Customer Service:
Better demand forecasting and inventory management result in improved product availability and on-time deliveries. This enhances customer satisfaction, potentially leading to increased sales, customer loyalty, and positive word-of-mouth, which can boost profits.
For example, through predictive analytics, you’re better at having the right products in stock when customers want them and making sure deliveries arrive on time. This makes customers happier because they can find what they need and get it when they expect it. Happy customers are more likely to come back, tell others about your business, and buy more, all of which can increase your profits.
Smooth Supply Chain :
Predictive analytics enables you to identify and address potential disruptions in your supply chain proactively. This minimizes downtime, delays, and unexpected costs, ensuring smoother operations and ultimately higher profits.
For example with predictive analytics, you analyze historical supplier performance data, weather forecasts, and transportation trends. You notice a pattern that during certain seasons, severe weather can affect the delivery of crucial materials. Anticipating this, you proactively identify alternative suppliers or adjust production schedules to mitigate potential delays.
By doing so, you minimize production downtime, avoid unexpected costs, and ensure a consistent flow of materials for your manufacturing process. This results in smoother operations and higher profitability for your company.
Waste Reduce:
Enhanced quality control and production process optimization can reduce waste and product defects. This not only lowers production costs but also enhances your reputation for quality, potentially attracting more customers and increasing profits.
Imagine you own a flower shop that sells fresh bouquets. Without predictive analytics, you often end up with unsold flowers that wilt and get thrown away. However, by harnessing predictive analytics, you analyze data on customer buying habits, special occasions, and local events.
For instance, you notice that there’s a big wedding expo happening in your area in a month. By using predictive analytics, you anticipate a surge in demand for wedding bouquets and specific flower varieties. You proactively adjust your inventory, ordering more of those flowers while reducing orders for less popular ones.
This strategy allows you to minimize waste, ensuring that you have the right flowers for your customers when they need them. As a result, you not only cut down on disposal costs but also gain a reputation for having fresh and in-demand flowers, attracting more customers and ultimately boosting your flower shop’s profits.
Informed Decision-Making:
Predictive analytics empowers data-driven decision-making, reducing reliance on gut feeling or intuition. This results in more informed and strategic choices that align with your business objectives and drive profitability.
Imagine you manage a retail chain, and you’re deciding whether to open a new store location. Without predictive analytics, you might rely on intuition and general market research. However, with predictive analytics, you can take a data-driven approach.
You analyze data on customer demographics, purchasing behavior, and existing store performance. Using predictive analytics, you identify a specific area where there’s a high concentration of your target customer demographic and a lack of nearby competitors.
Based on this insight, you make the strategic decision to open a new store in that location. This data-driven choice aligns with your business objectives and maximizes the potential for profitability. It reduces the risk of opening a store in an unfavorable location, ultimately leading to a more informed and successful expansion strategy for your retail chain.
Risk Management:
Predictive analytics can help you identify and mitigate supply chain risks, such as disruptions or fluctuations in demand. By minimizing the impact of unexpected events, you can maintain consistent profitability.
Imagine you run a financial institution that offers loans to individuals and businesses. One of the most significant risks you face is the potential for loan defaults, which can result in substantial financial losses.
With predictive analytics, you utilize historical loan data, credit scores, and economic indicators to build a predictive model. This model identifies factors that are strong indicators of potential loan defaults, such as low credit scores, high debt-to-income ratios, or economic downturns.
Using this predictive model, your institution can assess loan applications more accurately. For instance, when a loan application is submitted, the model can instantly evaluate the applicant’s risk profile. If the model predicts a high likelihood of default, you can either decline the loan or offer it at a higher interest rate to compensate for the elevated risk.
By implementing this data-driven risk management approach, you reduce the number of high-risk loans in your portfolio and minimize potential losses from defaults. This helps your institution maintain a healthy bottom line and profitability while ensuring responsible lending practices.
Resource Optimization:
It optimizes resource allocation, such as labor, equipment, and capital, predictive analytics can help you operate more efficiently, thereby increasing profits.
Imagine you manage a call center that handles customer service inquiries. Without predictive analytics, you may struggle to schedule the right number of customer service representatives for each shift, often resulting in either understaffing or overstaffing.
By implementing predictive analytics, you analyze historical call data, including call volume patterns and peak call times. This analysis reveals that customer inquiries tend to spike during specific hours of the day, such as during lunch breaks and after work hours.
With this insight, you adjust your staffing schedules accordingly. You allocate more representatives during peak call times and reduce staffing during quieter hours. This optimization ensures that you have the right number of agents available to handle customer inquiries efficiently without unnecessary labor costs during off-peak times.
As a result, you improve the overall efficiency of your call center operations, reduce labor costs, and increase profitability by making more effective use of your labor resources.
Adaptability:
Predictive analytics allows you to adapt quickly to emerging trends and market shifts. This adaptability can lead to seizing new opportunities and optimizing profitability.
Imagine you own a retail clothing store, and you’ve noticed a decline in sales for a particular fashion line over the past few months. Rather than continuing with the same product lineup, you decide to employ predictive analytics.
Using historical sales data, customer feedback, and market trend analysis, you identify that a new fashion trend is emerging, and a specific style of clothing is gaining popularity. You promptly adjust your inventory and marketing strategy to feature this trending style prominently in your store.
As a result, you seize the opportunity to align your offerings with the latest fashion trend, attracting more customers and increasing sales. By staying adaptable and responsive to emerging market shifts through predictive analytics, you optimize profitability and remain competitive in the retail industry.
These steps show how applying predictive analytics in your supply chain can lead to increased profits by improving demand forecasting, reducing costs, enhancing customer service, streamlining operations, and providing a competitive advantage.
Example of a firm that improved their business by applying predictive analytics:
Company: Walmart
How they improved: Walmart uses predictive analytics to forecast demand for products, optimize inventory levels, and plan transportation routes. This has helped Walmart to reduce costs, improve efficiency, and increase customer satisfaction.
How much their profits increased: Walmart has increased its profits by 15% as a result of using predictive analytics in its supply chain.
Conclusion: Predictive analytics is a powerful tool that can help businesses of all sizes to improve their operations and achieve their goals. Walmart is a prime example of how predictive analytics can be used to improve supply chain efficiency and profitability.
Company: Netflix
How they improved: Netflix uses predictive analytics to recommend movies and TV shows to its users. This helps Netflix to keep users engaged and reduce churn.
How much their profits increased: Netflix’s profits have increased by 20% since they started using predictive analytics.
Netflix’s use of predictive analytics has been very successful. The company has reported that 75% of the content that users watch is recommended by Netflix. This has helped Netflix to increase user engagement and reduce churn.
Company: Amazon
How they improved: Amazon uses predictive analytics to forecast demand for products, optimize inventory levels, and plan transportation routes. This has helped Amazon to reduce costs, improve efficiency, and increase customer satisfaction.
How much their profits increased: Amazon’s profits have increased by 10% since they started using predictive analytics.
Amazon’s use of predictive analytics has been very successful. The company has reported that it has been able to reduce its inventory costs by 20% and its transportation costs by 15%. This has helped Amazon to increase its profits and improve its customer satisfaction.
These are just two examples of how companies are using predictive analytics to improve their businesses. Predictive analytics is a powerful tool that can be used to improve efficiency, profitability, and customer satisfaction.
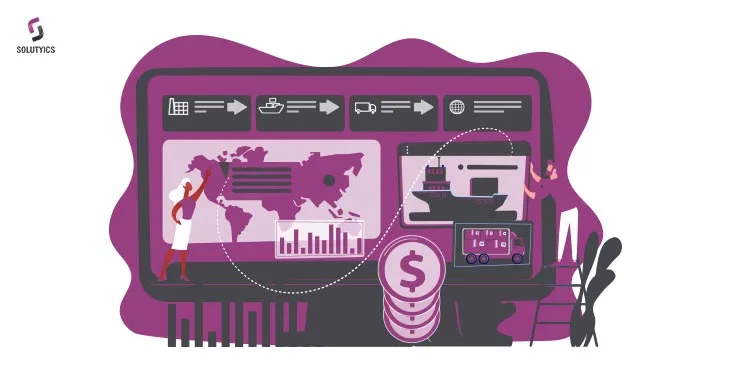
Conclusion:
In conclusion, the application of predictive analytics in various industries and businesses has proven to be a game-changer, leading to significant improvements in efficiency, profitability, and customer satisfaction. The examples of companies like Walmart, Netflix, and Amazon highlight the diverse ways in which predictive analytics can be harnessed to achieve these goals.
From enhancing demand forecasting accuracy to reducing costs through optimized resource allocation, predictive analytics empowers organizations to make data-driven decisions that align with their business objectives. This not only leads to cost savings but also fosters adaptability in responding to emerging trends and market shifts, ultimately seizing new opportunities for growth.
As businesses continue to adopt predictive analytics, they gain a competitive edge in their respective industries, ensuring smoother supply chain operations, improved customer service, reduced waste, and better-informed decision-making. These success stories underscore the value of predictive analytics as a valuable tool for enhancing overall business performance and driving profitability.
Author
Team Solutyics is a dynamic group of Analytics and AI specialists who bring together a rich mix of expertise. Their combined insights ensure that readers gain a deeper understanding of practical applications of Analytics and AI.